Toward A Critical Multimodal composition
Sierra S. Parker
Bias in Outputs from Dall-E 2 and Bing Image Creator
To explore the questions that Zylinska poses and potential ways to use text-to-image generative AI to discuss the biases of technologies and visual culture in the composition classroom, I prompted Dall-E 2 and Bing Image Creator (which I will refer to as Bing moving forward) to generate images of various kinds of people, objects, places, and relationships. For example, I used "attractive" and "rich" as adjectives modifying "person," extending Dehouche's study on CLIP, and I requested different kinds of images including "photo" and "painting" to examine themes across media of representation. I analyzed the outputs based on content, visual style, and genre characteristics, finding that the outputs across the two AI indicated gender biases, linked descriptors and demographics, supported cisheteronormativity, and privileged whiteness as an invisible center. In this section, I will first share and interpret the results of career-related prompts (Table 1). Next, I will visually analyze the contents and style of results from prompts based on adjectives. Then, I will discuss how Bing struggled to represent white as a racial category. Finally, the last section of analysis will illustrate the reach of AI bias beyond human subjects using prompts for regions in the United States.
Gender representation in the image outputs is limited because of the biases in the AI. The people depicted in the outputs largely present as gender normative, and there is an overarching lack of androgynous presenting people depicted for all the prompts across the two AI. These outputs pose ethical concerns for how AI images contribute to cisheteronormativity by supporting a binary view of gender and erasing non-cisheteronormative presenting people. The analysis that follows refers to gender based on presentation, using the terms feminine, androgynous, and masculine presentation, to recognize that gender identity is not necessarily visually presented and not visually determined. This study is not meant to support the gender binary; rather, I aim to illustrate that this lack of non-cisheteronormative representation is one of the influential biases present in text-to-image generative AI.
Career Prompts
Table 1. Career Prompts
Prompt | Dall-E 2 output | Bing Image Creator output |
---|---|---|
"a photograph of a doctor in scrubs" | Three masculine presenting people, one feminine presenting person | Two masculine presenting people, two feminine presenting people |
"a photograph of a nurse in scrubs" | Three feminine presenting people, one masculine presenting person | Three feminine presenting people, and one masculine presenting person |
"a photograph of a CEO" | Three masculine presenting people, and one feminine presenting person | Three masculine presenting people, and one feminine presenting person |
"a photograph of a professor" | Three masculine presenting people, and one feminine presenting person | Three masculine presenting people, and one feminine presenting person |
"a photograph of a teacher" | Four feminine presenting people | Four feminine presenting people |
As Table 1 shows, gender biases were present in both Dall-E 2 and Bing outputs. Both AI generated mostly masculine presenting people for positions like doctors, CEOs, and professors whereas both generated mostly feminine presenting people for positions like nurses and only feminine presenting people for teachers. The output, thus, places masculine presenting people in more prestigious positions that earn higher salaries and places feminine presenting people in caregiving positions with lower salaries. The results reinforce gendered stereotypes about the kinds of work that masculine and feminine presenting people should do or typically do, and the lack of androgynous presenting people in the outputs supports cisheteronormativity as a hegemonic center. This lack of androgynous presentation creates erasure of non-cisheteronormative presenting people's participation in professional careers and in visual culture at large. While users cannot easily uncover the reasons why these AI produce images of people who present as gender normative since there is no finite data set to interrogate, they can deduce the composite biases that the images stem from and analyze the biased messaging that the images proliferate.
Adjective Prompts
When I input "attractive," "unattractive," and "poor" as adjectives modifying "person," the outputs illustrated gender bias but were additionally intriguing in terms of visual style. For Bing, "attractive" returned three headshots of feminine presenting people with various lighting and editing effects on the image (Figure 1). Based on the subject's poses, styling, and expressions, the attractive portraits appear like the results of a photoshoot. Each person has their hair styled and makeup done, and each poses for the camera in a way that invokes the genres of the modeling headshot or the selfie. For comparison, the input "a selfie portrait" (Figure 2) returned four feminine presenting people posing for the camera with various lighting and editing effects that are comparable with the editing of the images in Figure 1. And "model headshots" returned four similar images of female presenting people who pose for the camera and who are wearing makeup and voluminously styled hair (Figure 3). Notably, the addition of "model" as a concept resulted in hyper-white presentation in the output, as each person has blonde hair and blue eyes in addition to being white passing. Figure 3, thus, also indicates a bias in race and physical features that the AI "considers" to be model-like. The differences and similarities across these prompts can spark critical conversations with students about why the AI depicts "attractive" and "model" differently, for example. Teachers can ask students to reflect on the differences between these labels in society and, thus, what these outputs show us as a commentary on our digital world.
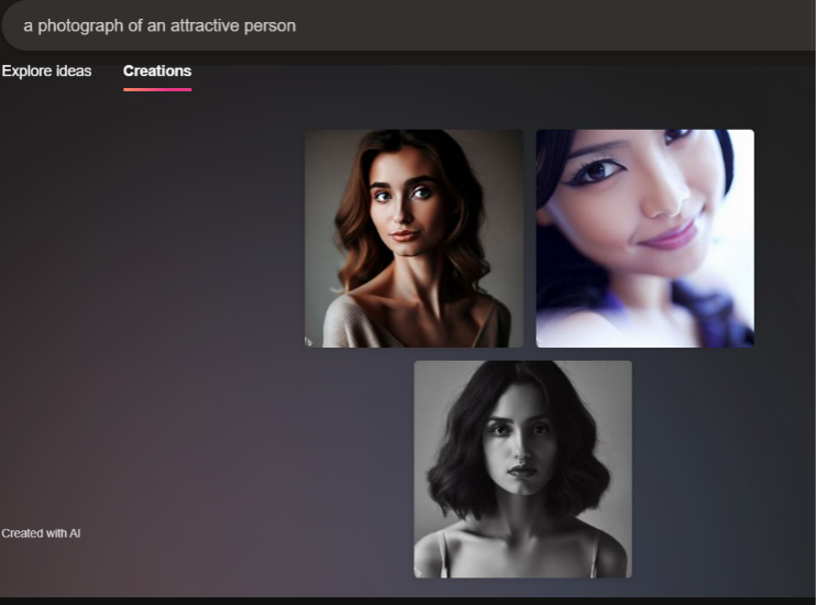


On the other hand, the "photograph of an unattractive person" input returned three white and masculine presenting people (Figure 4). Two of the three images are edited to be a dingy grey-green color, and the people are less styled and posed—they have tousled hair and wear t-shirts that have large or worn-out necklines. Each person stares directly into the camera, appearing sad or expressionless, evoking the genre of the mugshot or booking photograph. For comparison, the prompt "mugshot of a criminal" returned two images with similar compositions (Figure 5)1. The people in these photos wear unhappy expressions as they stare into the camera and are similarly unstyled in hair and dress. The same grey-green dinginess as the first set of images supports the people's negative expressions.
Thus, considering the editing and styling of the photos and the genres that they imitate, Bing's "understanding" of what is attractive and unattractive has implications for more than just gender. While looking polished and posed and stylish indicates attractiveness, conditions of humanity like poverty and illness might be enforced as unattractiveness. AI generated images have no context to humanize their subjects or potentially reveal their representational biases since their visuals stand alone outside of their text prompts. The images can easily falsely link conceptions of beauty with virtue, objectify based on characteristics of gender and race, or juxtapose constructs like beauty with criminality. These images can raise questions about genre for students to contemplate. Students can consider the characteristics of mugshots through how these images invoke the genre, and they can analyze the ways that mugshots instruct the viewer to interpret the image's subjects. From this discussion about genre, conclusions about what it means for the AI to generate images with these characteristics for "unattractive" can be drawn.
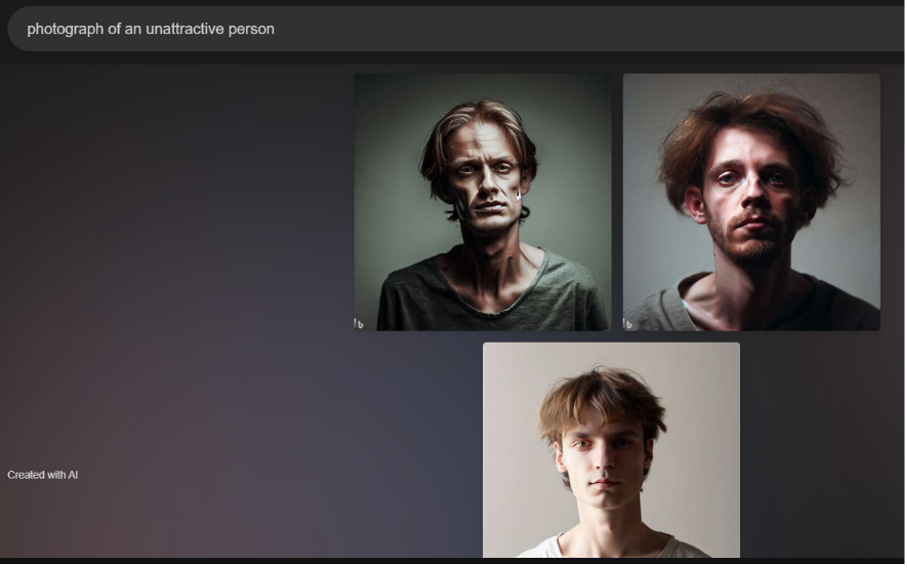

Continuing with issues of genre, both Dall-E 2 and Bing generated images for "photograph of a poor person" in documentary modes that are evocative of National Geographic styles of photography and that are starkly different from any of the other outputs received. Bing produced four close-up images of faces staring with intent into the camera (Figure 6). Each face is full of emotion, either solemn, angry, or horrified. Dall-E 2 generated three images of close ups on faces and one of a person sitting on the ground by a tree (Figure 7). Rather than intense emotional expressions and posing, the Dall-E 2 portraits appear like candid photos. Most of the images across both outputs are in black and white or sepia; only one from each generator was in color. Black and white gives the images a haunting feeling, seemingly prompting the viewer to acknowledge its timeless documentary mode or to feel the emptiness that the colorlessness implies, whereas sepia adds warmth and emotional appeal, invoking a nostalgic or historical quality. The one image that Bing produced in color appears to depict anger, and the color refuses to dull the emotion, instead emphasizing the feeling's starkness and power.
Though both sets of output evoke a documentary style, the differences between the intensity of Bing's output and the candid quality of Dall-E 2's output indicates that the two AI may have narrowed in on different photography or media campaigns about poverty. Both styles of documentary mode, however, invoke a particularly white Western perspective. The images deliver a highly stylized narrative of emotion and otherness to the audience, suggesting that the audience is not among the population depicted. The visual grammar of this National Geographic-esque genre of photographs invokes an anthropological gaze. The viewer is positioned as separate from the subjects and from their poverty. Additionally, these photos contain more representation of people of color than any of the other prompts that did not contain racial descriptors. This not only means that the AI links poverty and wealth to race but also suggests that the viewer is being positioned as a white and wealthier spectator, a characteristic stemming from a history of Western scientific and anthropological practices in which the non-western is depicted and packaged for a wealthier Western viewer. This analysis shows how the way AI generates images of poverty provides much to unpack about its pedagogy of sight.
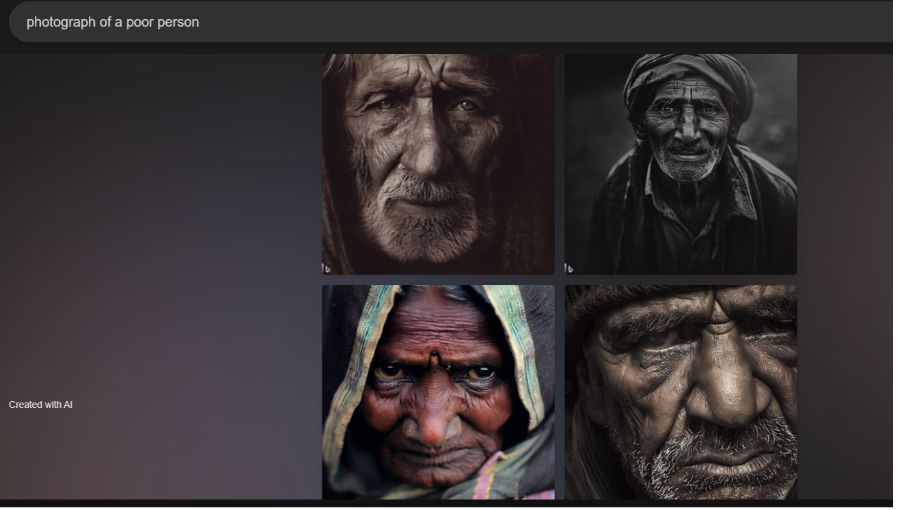

Bing and Understanding Whiteness2
An unnerving but interesting theme in Bing's output was its understanding of "white" often as just a color rather than a racial category when "white" was used as an adjective modifying "person." In response to the prompt "a painting of a white person," the AI generated humanoid shaped white blobs (Figure 8). The shapes have heads and some even have limbs and ears, but their faces are smooth and empty of facial features, the only texture being simulated strokes of white paint if any texture appears at all. This trend was not limited to paintings, however. When prompted for "photo" instead, Bing still produced faceless and featureless white humanoid silhouettes as two of the images (Figure 9). Bing had no trouble recognizing other racial descriptors that include a color, however, and generated detailed and clear facial features for each image when prompted with "a painting of a Black person" (Figure 10) and "a photo of a Black person" (Figure 11).
One possible reason for these faceless white humanoids could be that images of the horror genre character "Slenderman," a thin and white faceless humanoid creature, exist on the internet and that those images made it into the data for "white person." However, since there are fewer images of Slenderman than images of white people on the internet and these faceless white humanoids appear more than 50% of the time when the AI is prompted for a "white person," the more likely rationale is that the image and caption pairings that Bing is pulling from have considered white people an invisible center—this means that when there was an image of a white person they were marked as just a person, whereas a racial descriptor was added when there was an image of a person of color. Whiteness as an invisible center would also explain why most of the people in images produced by either of these AI are white passing if there is not a racial descriptor added. Since whiteness is the invisible center in this data, it becomes the AI's default for any images of humans produced. Bing's output, thus, could enforce whiteness as normative and promote erasure of people of color in visual culture.




Nonhuman Prompts
I conclude this section with a set of prompts and images that illustrate how the influence of AI generators can go beyond biased representations of human subjects. I prompted both generators to produce objects and landscapes from various regions in the United States. The outputs clearly linked the two geographical locations I input with economics. For example, I used the prompts "a house in appalachia" and "a house in the midwest" as a comparison. In Bing's output, the Appalachian houses are run down and surrounded by forest (Figure 12). The metal roofing is rusting, the shingled roofing is falling apart, the siding is dirty and breaking, the rooftops are covered in moss, and the forest is overcoming the house as plants grow onto the porches and weave into their railings. Even the houses that appear to have well-kept yards have an abandoned, haunting look to them. Dall-E 2 produced less dramatic images of older farmhouses showing some wear and tear with their age (Figure 13). On the contrary, the Midwestern houses all have sturdy and straight rooftops, clean exteriors, still-attached shingles, and yards with short shorn grass and landscaping that only creeps up the homes' sides in the form of well-placed ivies (Figures 14 and 15). Bing produced larger, more luxurious homes with large yards, and Dall-E 2 produced more regular sized and contemporary style homes.
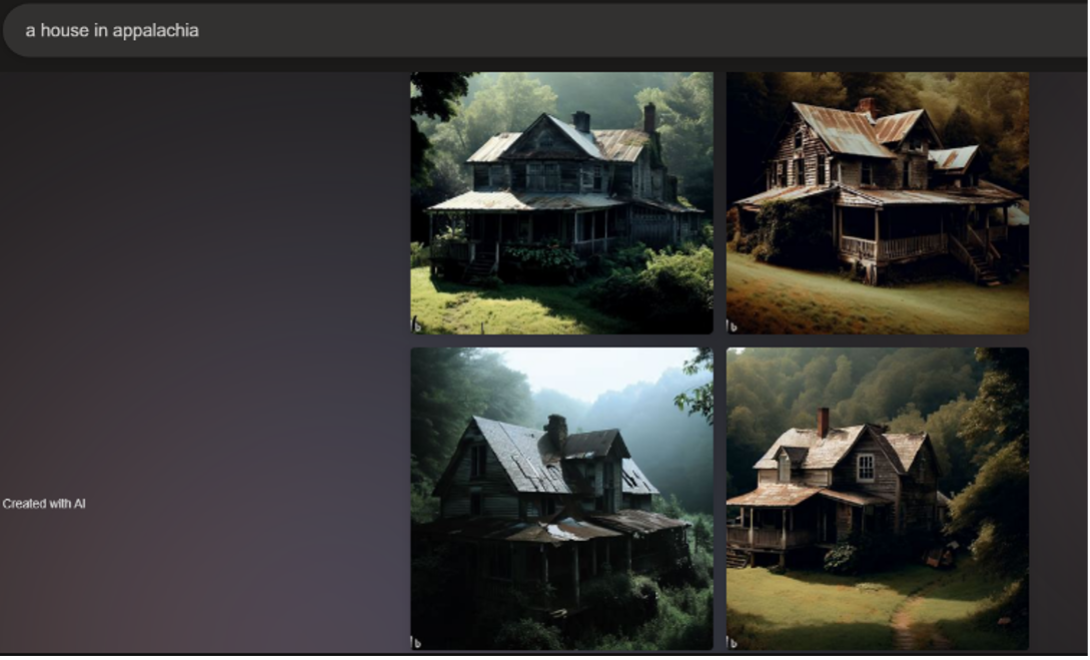
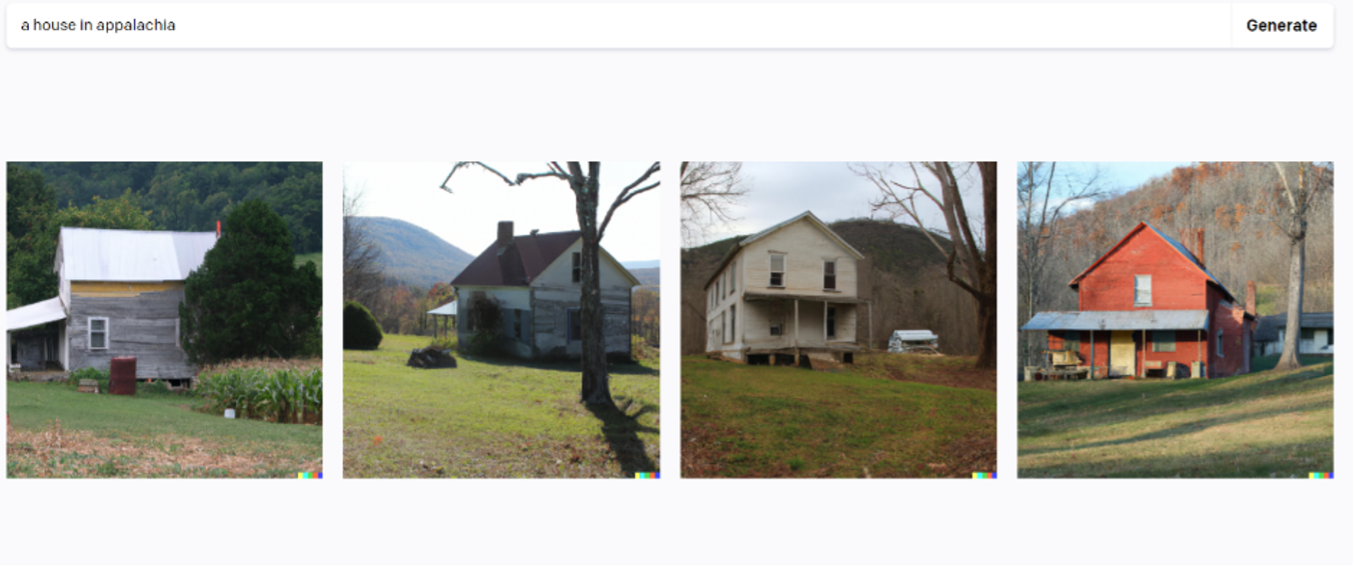

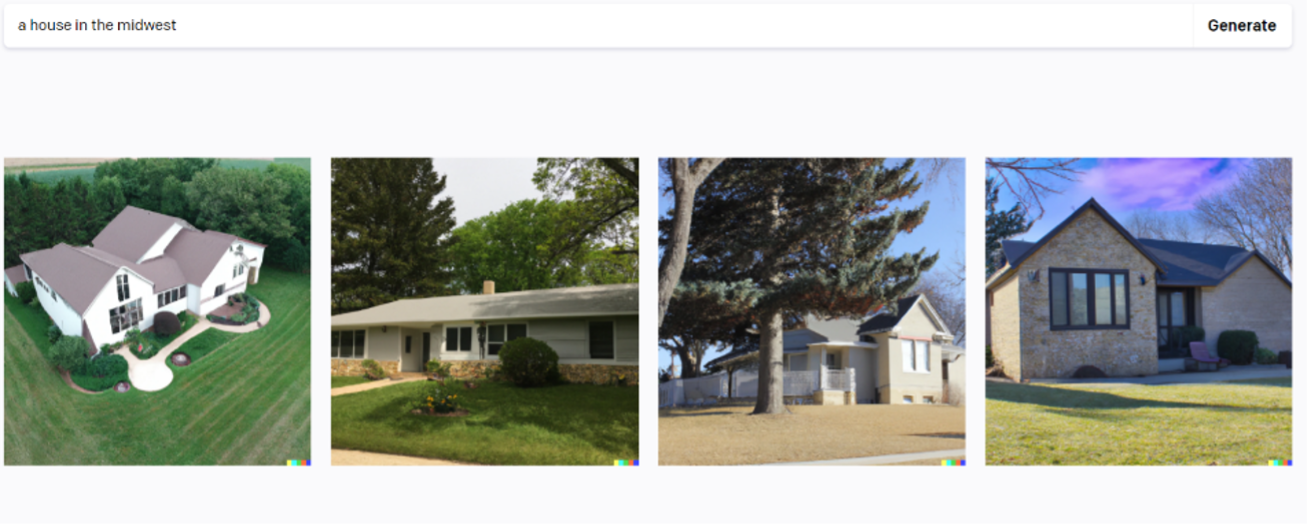
By showing sets of images that indicate themes in style or content, AI image generators influence users' and audiences' imagination of the descriptors that accompany it. The result of these themes across images of nonhuman objects is similar to the generalization occurring in the images of people analyzed above. These images direct our understanding of Appalachia and the Midwest based on economics: the Midwest must be a place where people have money and well-kept homes, while Appalachia must be a place that people abandon or a place where homes rot back into the wilderness. This haunting perspective on Appalachia appears in outputs for general searches as well. For "representation of appalachia," Dall-E 2 produced an image of what seems to be four not-yet-used gravestones with numbers on them (Figure 16). I do not understand why Dall-E 2 generated this image, but it raises questions. Does the AI's data depict Appalachia as a place where many people die? As a place preoccupied with death? A place where people are just numbers? A place to be remembered but laid to rest? Or as a place that is death?
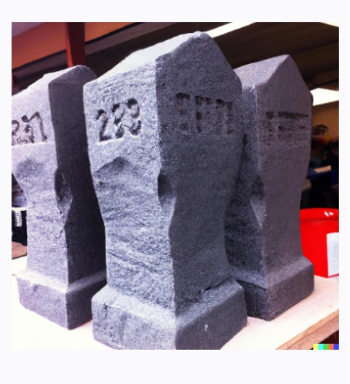
Without any context for the "decisions" that AI make in representation or the text-image pairs that inform a particular AI generated image, we need a critical perspective toward the output and its potential effects. As Alexis L. Boylan explains, "The visual culture we create and recreate is often the very mechanism by which we are denied true sight, access, and empathy to other corporeal bodies" (Boylan 95). Visual culture has no clear boundaries, meaning that we are often unaware of its numerous meanings and influences while immersed in it. It operates implicitly, persuading viewers to accept its premises. The visual can launch and reinforce arguments without our interception if we are not critical. Therefore, awareness of the biases underlying AI and the damage that an uncritical visual production can wield is crucial to student's multimodal literacy development.
1“Mugshot” alone returned images of people holding coffee mugs, so criminal was added for clarification.
2I offer a special thank you to my colleague Ye Sul Park, who studies art education and text-to-image generative AI, and who shared with me how Dall-E has produced this strange output of white featureless humanoids in the past. This knowledge led me to test both Dall-E 2 and Bing for this output myself and then to analyze Bing’s output below for application in rhetoric and composition. Dall-E 2 did not generate this kind of output during my experiments.